Keywords: Data Collection, Statistical Analysis and Synthesis, Qualitative Research
Aim: A research study conducted to interrogate ways in which online social media platforms infer behaviors, choices and other characteristics about social media users.
Note: This research study was approved by the Emily Carr Research Ethics Board and was in compliance with Tri-Council guidelines (TCPS2 2018) and Emily Carr University policies and procedures.
My Role: Co-Investigator
Abstract: Systems that make decisions about people based on their data, produce substantial adverse effects that can massively limit their choices, opportunities and life chances. The most predictive raw-material supplies come from intervening in our experiences to shape our behavior in ways that favor capitalists commercial outcomes. While using the internet, we give out personal data in the form of Name, Phone number and email address. But there is a lot of other information that gets recorded outside of our knowledge. This data is called data exhaust, which we do not give out voluntarily but can be inferred by the way we access the internet over a certain period. This data exhaust, when shared with third party advertisers and partners is what fuels the targeted ads industry and gives access to study user behavior.
This thesis research aims to validate the theory of interpreting behavioral data to make inferences about users. The research was conducted using information from the Co-Investigator’s (Aman Singh) LinkedIn account’s connections which were publicly available and had been consented to before sharing. Names of all connections were masked with the use of pseudo names. A written consent was taken from all connections prior to the start of the research to give them a free choice of opting out.
Methodology: Quantitative Data Collection & Content Analysis
Risks Associated: As a requirement of the research, the participant's area of work was included in the final data collection. This could lead to identification of the participants.
Risk Mitigation: Names of participants were anonymized and occupations were replaced with non-identifiable generic categories; if a participant was a Product Designer at Honda Motors, their area of work appeared as Automobile Industry.
Data Collection: The data collected from LinkedIn's View Activity section included:
1. what the connection had posted or shared on their wall
2. how old was this interaction or post
3. posts that my connections had liked, commented on or shared
4. hashtags used (if any)
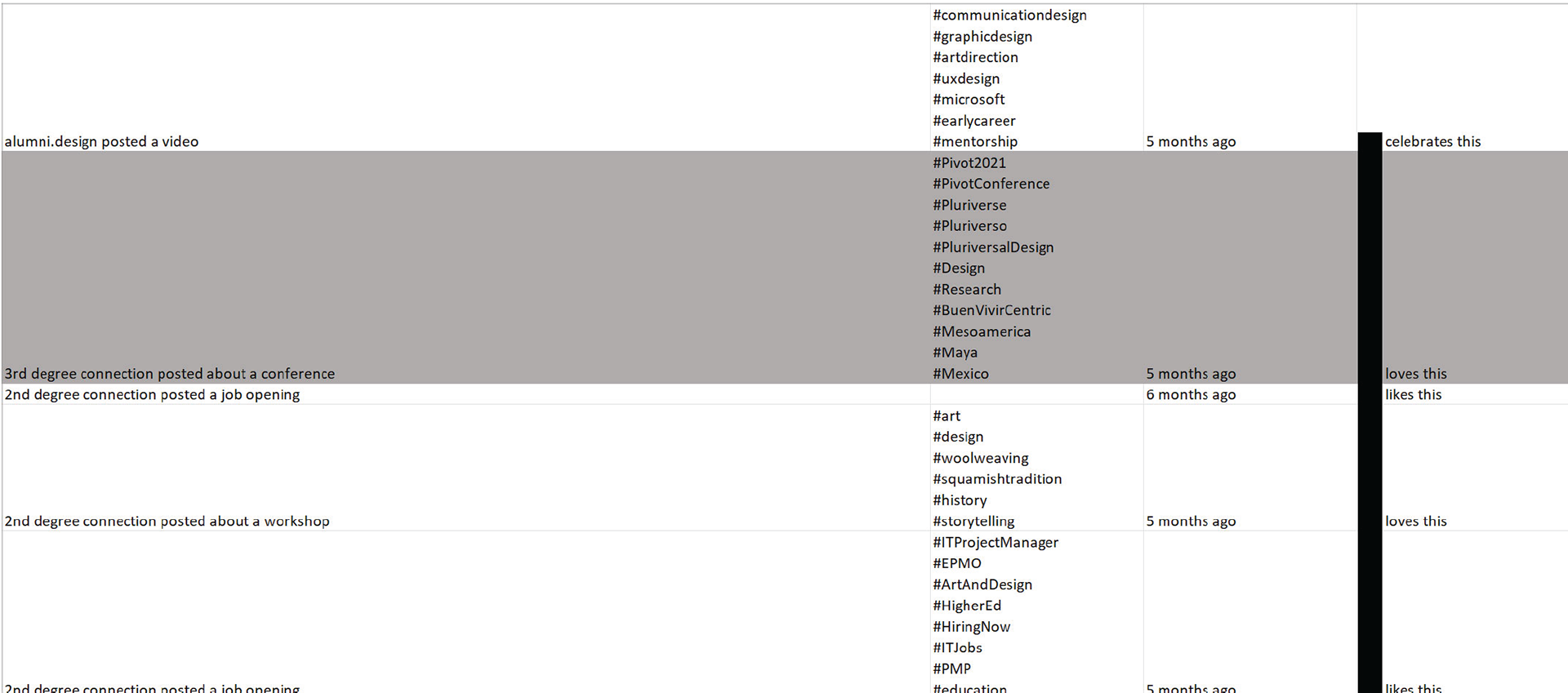
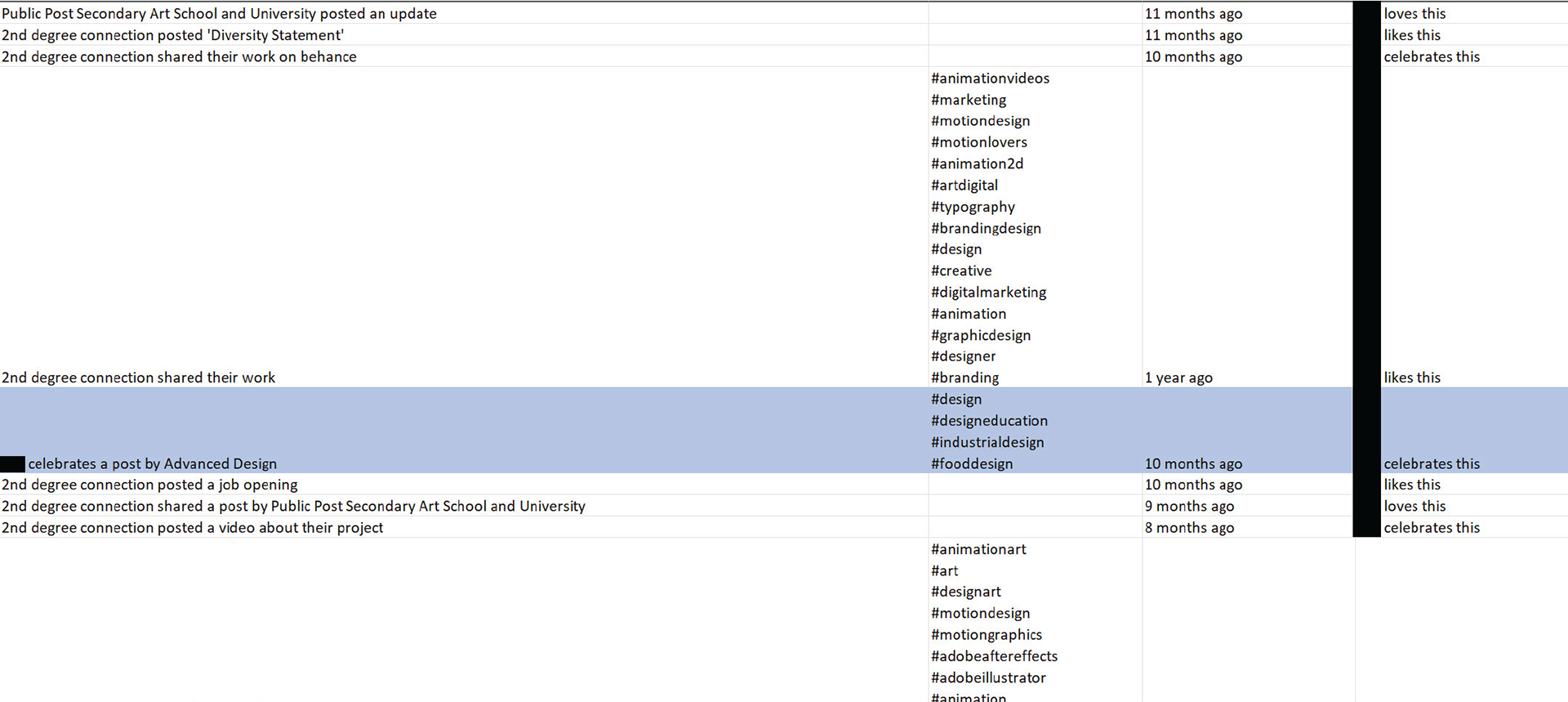
Data collection from LinkedIn
As these interactions were being documented, certain interaction patterns were recognized from the participants. For instance, one participant always liked a post and then shared it on their wall, one participant was always using the same set of hashtags on everything that they posted on LinkedIn. Some participants also had specific reactions to posts depending on who had posted it. A lot was also being learnt about the interaction habits of some of the participant's connections by going through their posts.
Apart from learning about the interaction habits of the participants certain changes that were being suggested by LinkedIn were also recognized. One of these was LinkedIn suggesting that ‘Open To Work’ be added to my profile even when I had recently updated my job just weeks before starting this project. It can be inferred that this suggestion was made since I was looking at many different profiles regularly while working on this project.
Conclusion: All these insights proved helpful in understanding how certain prediction algorithms might work for all their users on different online platforms. Based on the findings of this project, this is how I envision the inference cycle.
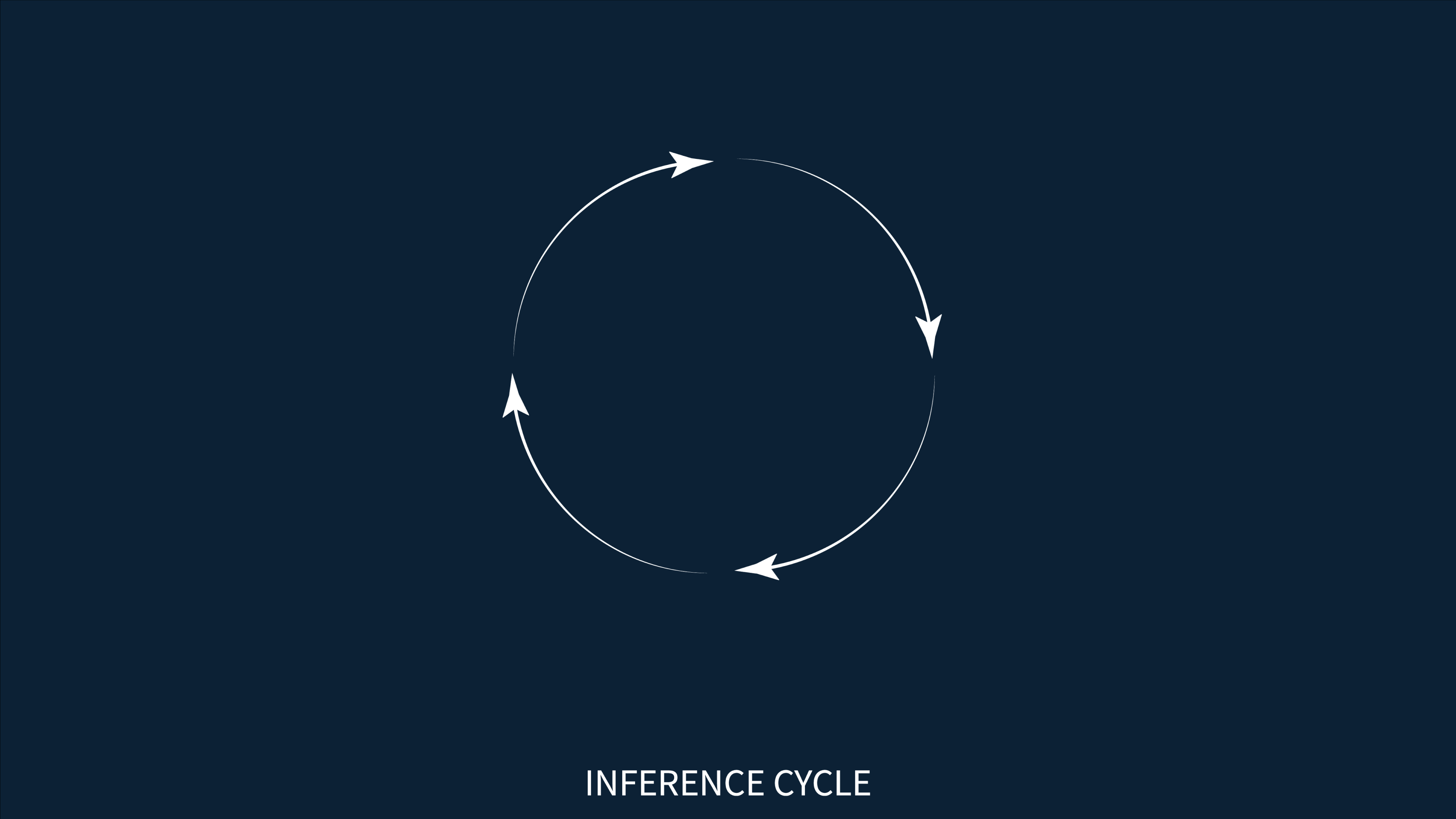